The Ultimate Guide to Image Labelling Tools for Businesses
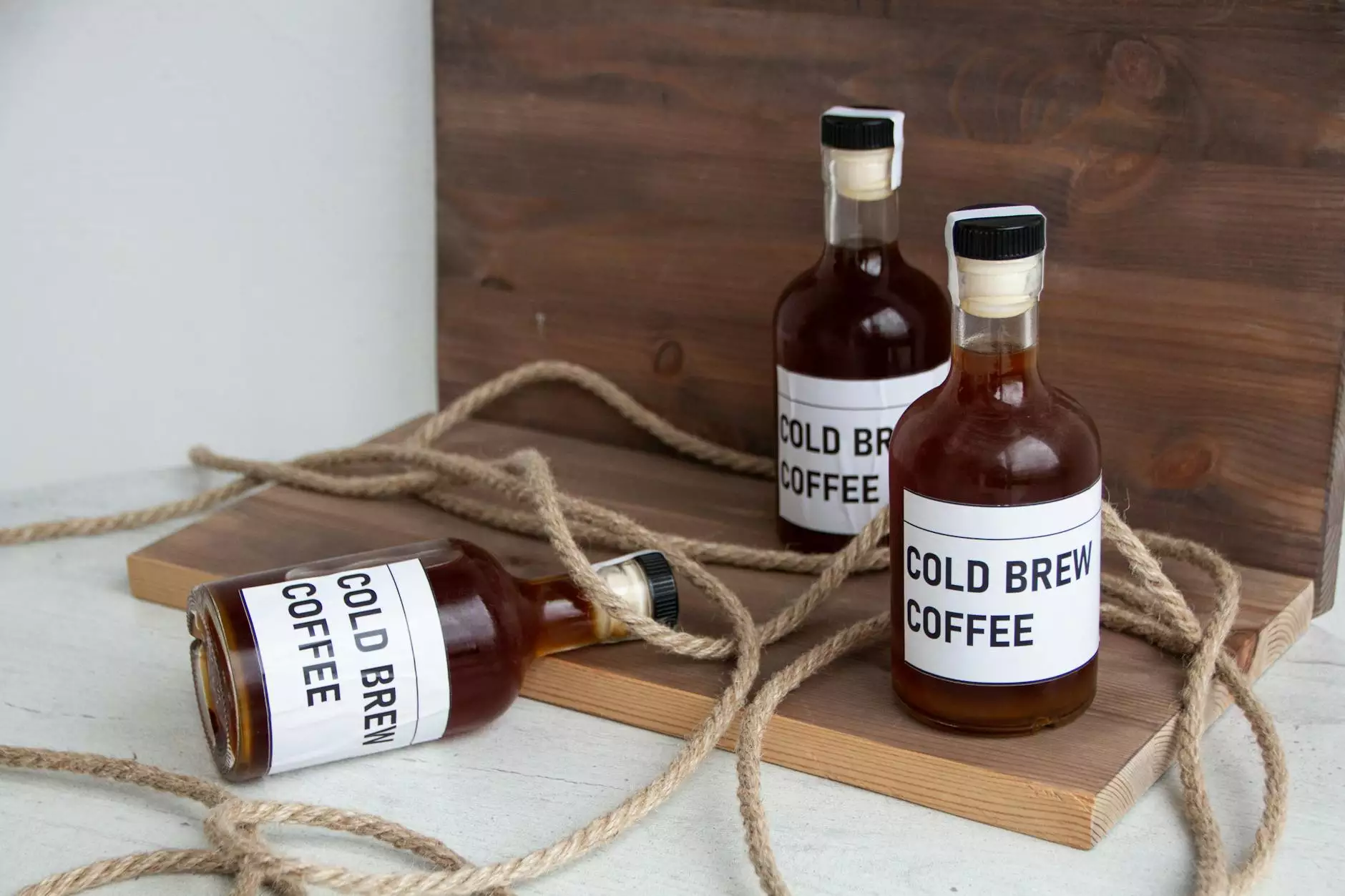
In the rapidly evolving world of data science and artificial intelligence, the need for accurate, efficient, and scalable data annotation solutions has never been more crucial. One such solution gaining prominence is the image labelling tool. These tools play a pivotal role in training machine learning models, offering businesses a significant edge in their respective industries.
Understanding Image Labelling Tools
Image labelling tools are specialized software applications designed for annotating images with metadata or labels. This process is integral to creating datasets that machine learning algorithms can learn from. Without proper labelling, AI systems may struggle to recognize patterns, leading to less effective outcomes in various applications, ranging from healthcare diagnostics to autonomous driving.
Why Do Businesses Need Image Labelling Tools?
As businesses increasingly rely on advanced analytics and AI, the need for high-quality annotated data becomes essential. Here are several compelling reasons why integrating an image labelling tool is beneficial:
- Enhanced Data Quality: High-quality annotations improve the performance of machine learning models, leading to better prediction accuracy.
- Scalability: Automated image labelling tools can handle large datasets efficiently, supporting the growing data needs of businesses.
- Time Savings: Automation reduces the amount of time required to label images, allowing teams to focus on higher-value tasks.
- Cost-Effectiveness: By streamlining the labelling process, businesses can reduce operational costs associated with data preparation.
Features of an Effective Image Labelling Tool
Choosing the right image labelling tool involves considering several key features that enhance user experience and output quality. Here are the essential features to look for:
- User-Friendly Interface: A simple, intuitive interface ensures that team members can easily add labels without extensive training.
- Support for Multiple Formats: The tool should support various image formats because businesses often handle different types of visual data.
- Collaboration Features: Multi-user functionality allows teams to work together on projects, facilitating real-time feedback and updates.
- Integration Capabilities: The ability to integrate with other software and platforms can streamline workflow, making data export and analysis easier.
Types of Image Labelling Tasks
Image labelling encompasses various tasks, each serving different purposes within the context of machine learning. Some common types of tasks include:
- Bounding Box Annotation: Used primarily in object detection tasks, this method involves drawing rectangles around objects of interest in images.
- Semantic Segmentation: This process assigns a class label to every pixel in the image, making it suitable for applications requiring detailed scene understanding.
- Image Classification: A straightforward task where entire images are labeled with a single category. This is crucial for general categorization tasks.
- Landmark Annotation: This technique identifies specific points (landmarks) on objects, useful in facial recognition and medical imaging.
How Image Labelling Tools Enhance Machine Learning Models
The quality and accuracy of data annotations directly impact the effectiveness of machine learning models. Using an image labelling tool can result in several benefits:
1. Improved Recognition: Well-labeled images help machines learn to recognize patterns and objects more effectively, enhancing overall model performance.
2. Reduced Errors: Automated tools minimize human errors during the annotation process, ensuring a higher quality dataset.
3. Faster Training Times: Accurate annotations lead to more efficient training processes, allowing models to learn quicker and more effectively.
The Role of Data Annotation Platforms
While different image labelling tools exist, they often come integrated into data annotation platforms that offer a wider range of services. Key aspects of these platforms include:
- Comprehensive Features: They often incorporate not just image labelling but also video and text annotation capabilities.
- Project Management Tools: Many platforms provide tools to manage entire data annotation projects, including progress tracking and deadline management.
- Reporting and Analytics: Advanced analytics features help teams evaluate the effectiveness of the labels and the overall quality of the dataset.
Choosing the Right Image Labelling Tool
When selecting an image labelling tool, businesses should consider the following aspects to ensure they choose the best solution for their needs:
- Specific Use Case: Different tools perform better depending on the type of project. Identify your requirements and choose accordingly.
- Budget Constraints: Determine your budget for data annotation tools and find an option that delivers good value for your investment.
- Trial Versions: Many platforms offer trial periods; take advantage of these to assess usability and features before making a commitment.
Integrating Image Labelling Tools into Your Workflow
The integration of an image labelling tool into your existing workflow can be seamless if done correctly. Here are practical steps to follow:
- Identify Key Stakeholders: Engage with team members who will be using the tool to gather their input on requirements and preferences.
- Establish a Data Strategy: Develop a clear plan for how data will be handled, from annotation through to model training and deployment.
- Train Your Team: Provide training sessions to help your team become proficient in using the new tools effectively.
Real-World Applications of Image Labelling Tools
Image labelling tools find applications across various industries, driving innovation and efficiency. Some notable examples include:
Healthcare
In the healthcare sector, annotated images are crucial for developing diagnostic tools. For instance, medical imaging requires precise labelling to train AI systems for disease detection.
Autonomous Vehicles
Self-driving cars rely on extensive image data to navigate safely. Image labelling tools are essential for annotating road signs, pedestrians, and obstacles, ensuring the algorithms learn effectively.
Retail and E-commerce
In retail, image labelling assists in product recognition systems. By annotating product images, businesses can enhance customer shopping experiences through better search algorithms.
Future Trends in Image Labelling
The landscape of image labelling is continually evolving with the advancements in AI and machine learning. Some trends to watch include:
- AI-Driven Annotation: Future tools may utilize AI to assist in the annotation process, automatically suggesting labels and drastically reducing manual work.
- Increased Collaboration: As remote work becomes more prevalent, tools that enhance team collaboration during the annotation process will gain importance.
- Real-Time Annotation: The demand for real-time processing of image data will drive innovations in tools that allow quicker labelling.
Conclusion
In summary, the necessity for effective and reliable image labelling tools for data annotation cannot be overstated. With the right tools and strategies, businesses can transform their data handling processes, leading to more accurate machine learning outcomes and driving efficiencies across operations. Leveraging advanced data annotation platforms will not only save time and resources but will also provide a competitive advantage in today's data-driven market.
For more insights into how to harness the power of image labelling tools for your business, visit keylabs.ai today.